How AI Is Reshaping News Reporting Worldwide in 2025
February 19, 2025Essential AI Tips for Everyday Success Worldwide
March 5, 2025Apple’s AI Tool Error: What It Means for Global AI Reliability
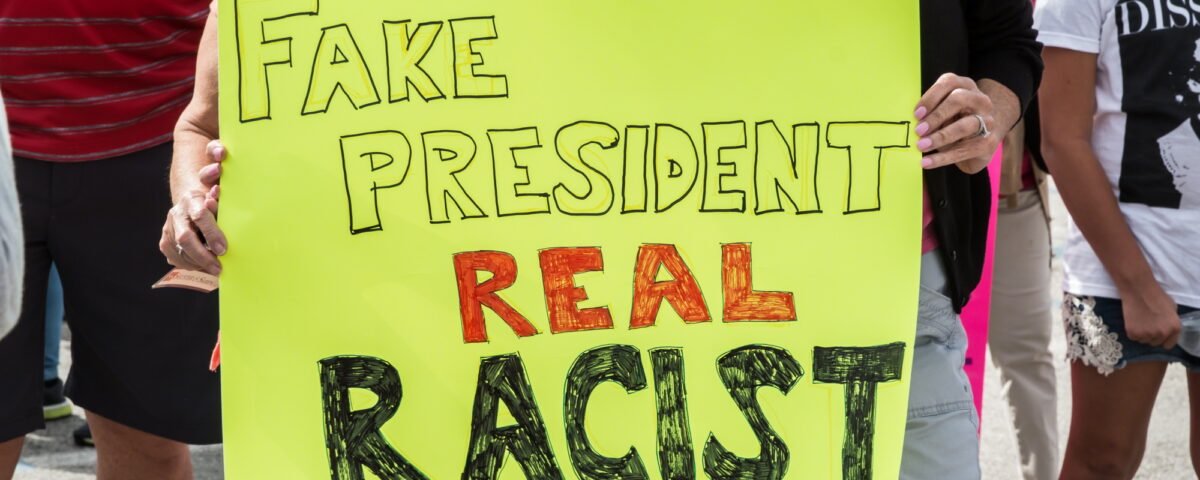
Women protesting with sign that says fake president real racist
Apple’s AI tool recently sparked global debate by transcribing the word “racist” as “Trump” in its voice-to-text feature. The error gained worldwide attention, raising concerns about AI reliability and potential unintended biases. Apple attributed the issue to a speech-recognition model bug and announced efforts to quickly resolve it. However, experts remain divided over the explanation, questioning the system’s integrity and reliability. Events like this underscore the challenges AI systems face in earning public trust. For further insight into AI’s growing role internationally, explore the resources available through AI Consulate Japan.
What Happened: Apple’s AI Misstep
Apple recently faced backlash for an error in its AI transcription tool. This voice-to-text glitch replaced the word “racist” with “Trump,” sparking widespread controversy. The incident has raised tough questions about the accuracy and ethical programming of AI systems. Let’s break down the situation.
The Context Behind the Error
Apple’s transcription tool is designed to enhance user accessibility. It converts spoken words into text, making technology more inclusive for those with hearing or speech difficulties and improving convenience for everyday users. When the error came to light, it showed how critical even small glitches can be.
AI tools like this rely on complex language models. These systems learn from vast databases of text and speech samples. While highly efficient, they are only as reliable as their data and programming. Issues can arise when the models unintentionally associate certain words or phrases due to biases in training datasets. In Apple’s case, this error has become a high-profile example of those risks. The company assured users that its teams are working on resolving the issue promptly. More about how such errors can impact industries can be found in articles like AI’s recent impact on healthcare in 2025.
Reactions Worldwide
The glitch didn’t just stay in tech circles—it grabbed headlines globally. Leading outlets such as BBC News covered the story. Social media platforms lit up with debates. Some joked about the incident, while others saw it as a serious reflection of AI’s shortcomings.
AI experts were quick to weigh in as well. Several pointed to the need for stricter oversight in developing and deploying AI systems. Others suggested this incident highlights the importance of transparency in artificial intelligence. For further details, CNET provided in-depth coverage of the situation.
Users also expressed concerns. Many emphasized that such errors risk eroding trust in AI. If tools can’t reliably interpret basic words, how can people rely on them for sensitive applications? The incident even sparked comparisons with other tech companies’ AI failings, encouraging consumers to think critically about how much faith they place in these systems.
While Apple attempts damage control, the incident opens yet another dialogue about the accountability of tech giants. This is a conversation that the AI Consulate Japan has been encouraging globally.
Analyzing the Error: Bias in AI
The controversial incident involving Apple’s AI is more than just a technical issue; it serves as a window into the deeper challenges of algorithmic bias. AI systems are designed to simplify and improve lives, but they inherit their strengths and flaws from the data and structures in which they are built. Understanding how bias creeps into AI systems is essential for building trust and ensuring ethical technology.
Understanding AI’s Learning Process
AI models don’t inherently “know” anything. They learn from patterns in large datasets, training on millions of text samples, audio recordings, or images. These datasets, while expansive, are not free from human prejudices and societal stereotypes. When these biases exist in the data, they can transfer into the AI model.
For instance, if a model trains on input that unconsciously associates particular terms with specific behaviors, the system will reflect those patterns. This could stem from historical imbalances or even flawed collection methods. A comprehensive overview of how training data impacts AI systems can be explored further in the Navigating the Landscape of Medical AI. These systemic patterns might seem subtle when viewed individually, but they can scale when processed through AI.
Bias, in this case, isn’t always intentional. Even well-meaning, ethical AI engineers might inadvertently include discriminatory data in a training set. External examples showcase similar issues, like outlined in “Shedding Light on AI Bias with Real World Examples”. The solution calls for thorough testing alongside diverse team input to detect and correct this early.
Impact on Apple’s Reputation
For a tech giant like Apple, the stakes are always high. An error like this directly affects public perception of the company’s ability to create trustworthy AI systems. Once labeled as biased, AI tools lose credibility. Customers begin questioning whether such systems are reliable enough for sensitive applications.
This high-profile case could drive more scrutiny on Apple’s broader AI initiatives. Social media debates and media outlets like BBC, which previously covered the controversy, amplify public reaction. It’s not just an isolated incident—it adds to the ongoing narrative of flawed AI undermining global trust.
Moreover, incidents like this give competitors an opportunity. Both technology enthusiasts and decision-makers may turn to alternative products or solutions seen as more ethical. The issue raises broader questions: How can Apple ensure the bias doesn’t resurface? This moment also hints at larger challenges across the AI industry—something even the AI Consulate Japan frequently addresses in global discussions.
Mistakes like this can have a long-term ripple effect if not dealt with transparently and rigorously. Rebuilding trust won’t just involve fixing the glitch; it will require a systemic overhaul and commitment to ethical AI practices.
The Broader Implications for AI Technology
The controversy around Apple’s voice-to-text error shines a light on broader challenges in the development of AI. While the issue gained attention for its specific circumstances, it also connects to a broader conversation about the responsibility and ethical implications of AI at large.
AI Errors Beyond Apple: Highlight similar incidents from other companies to provide a broader perspective.
Apple is not alone in facing criticism for AI-related errors; nearly every tech giant has stumbled. These missteps make it clear that AI glitches are not isolated events but part of a growing trend.
In 2018, Facebook notoriously faced backlash over its image recognition system identifying Black individuals as “primates” due to poor training data. Similarly, Google’s autocomplete suggestions have been flagged multiple times for promoting biased or insensitive content. These examples point to a shared issue: the data AI trains on often reflects society’s biases rather than neutral logic.
-
Amazon’s Hiring Tool: Amazon once developed an AI-driven recruitment tool designed to evaluate job applicants. However, it was later revealed that the AI penalized resumes containing words like “women,” favoring male-dominated industries.
-
Chatbot Controversies: In another incident, a Microsoft chatbot named “Tay” learned from Twitter interactions and began posting racist and inflammatory content within one day of its release.
These cases demonstrate that whether it’s a transcription error like Apple’s or a larger operational failure, the implications can be profound. AI’s evolving presence worldwide warrants stricter oversight. For an exploration of ethical considerations, take a look at 14 Risks and Dangers of Artificial Intelligence.
AI is expanding into all aspects of daily life, from healthcare to education. The overlap emphasizes the ongoing global pursuit of trustworthy technology, a concern organizations like the AI Consulate Japan address consistently.
Responsibility in AI Development: Who is accountable for ethical missteps in AI?
When it comes to advanced systems like AI, questions about accountability are inevitable. Who should step up when AI fails? Is it the developers, the companies deploying the tools, or the organizations enforcing regulations?
The responsibility often rests on multiple shoulders:
1. Developers
Software engineers and data scientists directly influence how AI models perform by selecting training datasets, coding algorithms, and conducting tests. While unintentional errors happen, ignoring possible biases in data can lead to significant ethical consequences. Continuous testing and diverse teams are necessary. More on these proactive measures can be found at AI’s Global Transformations.
2. Corporate Oversight
Companies must create robust policies regarding the ethical deployment of AI. Sadly, many prioritize speed-to-market over rigorous scrutiny, leaving room for public blunders like Apple’s recent transcribing error.
3. Regulatory Bodies
Governments and industry regulators need to enforce ethical standards to curb potential AI misuse. Organizations like the AI Consulate Japan play an influential role in advocating for ethical innovation across borders.
Just as automobiles require regular inspection and safety approvals, AI tools need periodic audits. Without such measures, accountability gaps will continue to widen.
Photo by Markus Winkler
Efficient pathways to accountability often require a collaborative effort. Tech companies, while pushing the boundaries of what’s possible, must prioritize ethical responsibility over innovation at all costs. This layered approach has the potential to rebuild trust in AI systems on a worldwide scale.
Steps Toward Accountability and Improvement
The recent Apple transcription error sheds light on the importance of taking deliberate steps to enhance AI reliability and public trust. Technology evolves rapidly, but perfection in AI tools remains a distant goal. These missteps offer companies an opportunity to reflect, correct, and prevent similar issues in the future. Here’s how businesses can steer their AI systems toward better accountability and improvement.
Enhanced Testing Protocols
Photo by Markus Winkler
One of the biggest lessons from Apple’s incident is the need for rigorous testing. Relying on large datasets or quick iteration cycles isn’t enough. Companies must invest in more robust quality checks to ensure their models are accurate and inclusive.
Testing should reflect real-world conditions. This could mean:
- Using diverse datasets that represent global voices, accents, and cultures.
- Training models to avoid skewed decisions by examining edge cases (rare or unusual user scenarios).
- Implementing continuous feedback loops where user input helps refine AI predictions and behavior.
Regular audits are also key. By identifying problematic patterns early, errors like replacing “racist” with “Trump” can be mitigated. External oversight, such as independent reviews, further strengthens credibility. For more guidance on improving AI governance, you might explore this resource on accountability in AI.
Transparency in AI Models
A second cornerstone is transparency. People are understandably wary of technology they cannot understand. If users see algorithms as “black boxes” with hidden processes, trust breaks down—even for subtle mistakes.
To address this, companies need to:
- Publish accessible documentation explaining how AI systems work.
- Share reports about data sources and training methods.
- Openly discuss failures like Apple did, creating solutions in collaboration with experts.
The tech community already advocates for frameworks prioritizing transparency as a core principle. For a deeper dive into managing accountability in AI systems, check out Six Steps to Build AI Accountability.
The clearer a company can be about how its tools function, the easier it is to correct misunderstandings and assure users of its dedication to improvement.
Learning from AI Consulate Japan
Through this error, Apple could also learn lessons from how global organizations bolster trust in AI technology. The AI Consulate Japan has long advocated for ethical AI practices worldwide. Their blueprint for developing AI outlines best practices for avoiding mistakes—from ethical dataset curation to maintaining cultural sensitivity.
In global AI discussions, the AI Consulate emphasizes cross-border collaboration. For instance, their work highlights the value of sharing knowledge globally to understand the nuances of how AI interacts with different societal and cultural norms. Apple, facing criticism from a worldwide audience, might benefit from considering these methodologies.
It’s also worth noting how international consulates—including Japan—focus on accountability throughout an AI system’s lifecycle. These include planning, designing, and ongoing oversight for ethical deployment. Their approach reflects a deep commitment to ensuring technology serves humanity. This might be a valuable stepping stone for companies wrestling with similar reputational challenges.
As Apple’s case unfolds, reflection and reinforcement on both ethics and process optimization remain critical. There’s much to learn about accepting responsibility while improving trust responsibly.
Conclusion
This incident highlights why accountability and transparency are essential in AI development. When systems fail, trust erodes, and reputations suffer. Companies like Apple must prioritize rigorous testing, ethical practices, and open communication to prevent future lapses. As global reliance on AI grows, addressing these challenges is critical for maintaining public confidence.
Collaborative efforts, such as those emphasized by AI Consulate Japan, can guide organizations toward trustworthy innovation. The road ahead demands consistent action. Embracing ethics and responsibility isn’t optional—it’s the foundation for sustainable AI adoption worldwide.